Local governments across the country are starting to look to tech, big data and artificial intelligence for new answers to old questions. How can cities be most efficient with their limited resources? How can they ensure fairness towards individuals and groups of citizens? How can they improve safety, affordability, economic opportunity, and quality of life?
From public works to public health, the range of possible city applications for AI and complex algorithms is vast. But when we talk about using AI to fix potholes faster, get rats out of restaurants, and keep criminals off the street, we must recognize that even if the solutions to these problems are technically similar, the ethical risks vary widely. That difference, as the basis of a taxonomy of AI applications, provides a useful framework for evaluating potential AI applications.
AI applications have different levels of risk, defined as the likelihood of causing serious harm through discrimination, inaccuracy, unfairness or lack of explanation.
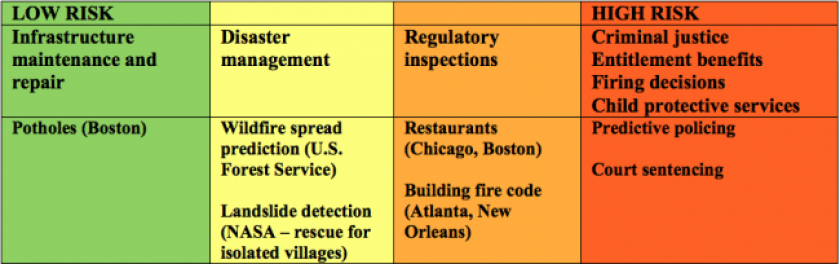
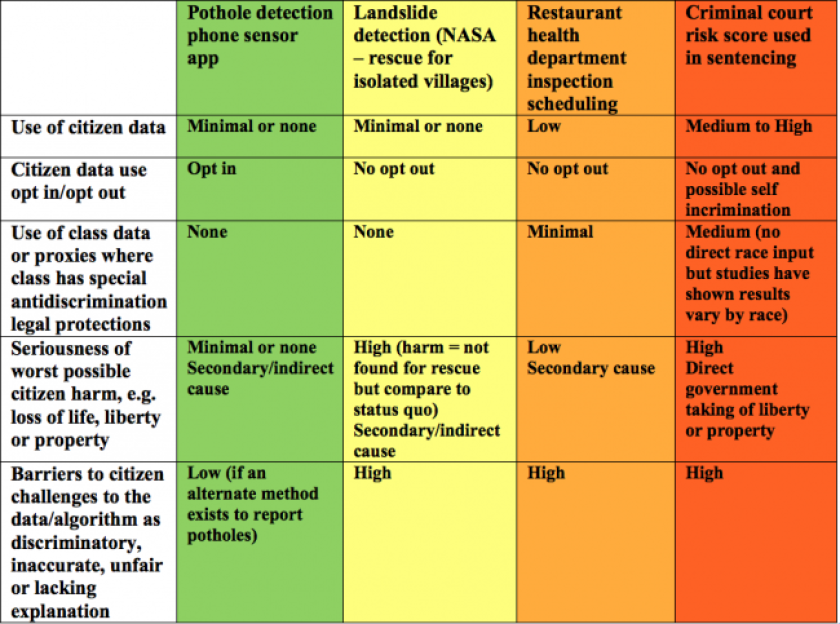
Decision makers may choose a different set of questions and risk factors or weight them differently than we did here, and they may come to different conclusions about an application’s AI ethical risk. These variations are predictable byproducts of political and cultural differences, but the most important thing is that cities start asking these questions and thinking carefully about the potential risks of AI. Moreover, they should ask them more than once, throughout the process of initial consideration, development (or procurement), deployment and evaluation.
If you're a city leader looking for a place to start with AI that's relatively safe, look to the green column on the far left side of the ethical risk continuum. Take one example there: directing public works resources — like filling in potholes — to neighborhoods where citizens use a city app probably skews to a certain demographic, but bumpier roads for some neighborhoods is a lesser potential harm than many other unwanted outcomes from AI. Moreover, there are ways to fix that problem. For instance, the selection bias could be ameliorated by maintaining a second method for directing crews: for example, using 311 calls or putting the app on city buses. As I heard one mayoral chief of staff say at a recent Project on Municipal Innovation meeting held by Harvard Kennedy School Ash Center and Living Cities, if you can't fix the potholes and keep the snow plowed, you can't get reelected. So maybe that AI pothole app is a pretty good idea.