One late night in June 2011, police patrolling Arlington Cemetery in northern Virginia stopped a suspicious individual making his way among the lines of veterans’ white gravestones. The man was no mere trespasser, but a much sought-after prize: the notorious sniper who had recently fired multiple rounds at the Pentagon, the Marine Corps Museum, and Marine Corps and Coast Guard recruiting stations.
Intercepting the shooter was no accident. His behavioral profile had been mapped using predictive analytics — statistics, renderings, and other data about the sniper’s previous shootings had been analyzed with a software model first developed for the U.S. Department of Homeland Security. On that night in Arlington, police caught the sniper in exactly the kind of location the model had predicted: near a place of military significance, with long lines of sight, and close to a major highway.
Predictive analytics of the sort that helped nab the Virginia sniper have a long history in crime prevention, dating to New York City’s fabled turnaround in the mid-1990s. Then-Police Chief William Bratton’s pioneering CompStat program was among the first to bring a data-based approach to crime reduction, quality of life improvement, and resource management. Bratton’s system began humbly — with coded pins stuck in maps to track crimes by neighborhood — but became a break-out success, credited with curbing citywide crime by 60 percent.
Today, the science of data analytics is light years beyond those nascent efforts from two decades ago. Computing power has doubled every 40 months since the 1980s, and with it, too, the ability to analyze vast quantities of data and predict future behavior.
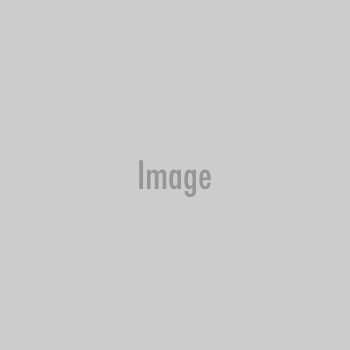
“We’re on the cusp of a revolution in law enforcement, one that’s transforming the very essence of police work,” said Colleen McCue, a veteran of the Richmond, Va., police department who, as a senior director at DigitalGlobe, oversaw the design of the data-based program used to catch the Virginia sniper. “Data analytics provide a management solution that people dream of finding, and rarely do — how to do more and to do more better, but with less.”
Deploying resources more efficiently, anticipating the behavior of criminals, boosting officer morale by ensuring support is nearby, estimating where pockets of crime will spring up before they do — data analytics deliver these and many other benefits, say McCue and other law enforcement experts.
Indeed, the cities that have adopted data-based approaches to crime prevention are seeing remarkable results.
California is experiencing a boomlet in crime-fighting success since the introduction in 2012 of PredPol, short for “predictive policing,” a data analytics software platform created by researchers at UCLA, Santa Clara University and UC Irvine.
PredPol anticipates where crimes are likely to occur in geographic prediction boxes as small as 500 feet by 500 feet. The Santa Cruz Police Department, which pioneered PredPol in 2011, reported a 27 percent reduction in burglaries that year and a 19 percent reduction in property theft by 2012. In Los Angeles, crimes were down 13 percent where PredPol debuted in 2012, compared with an increase of 0.4 percent elsewhere in the city.
“PredPol and other data analytics aren’t a substitute for good police work, they’re another tool police can use to elevate all their methodologies, including their own best on-the-ground instincts,” said George Mohler, the Santa Clara University professor who developed the PredPol algorithm. “The feedback we’re getting is that everyone, from police chiefs down to beat cops, is able to put data analytics into play quickly and effectively.”
The new technologies arrive at a time of dire need, as cities across the country confront municipal budget cuts that are forcing unprecedented downsizings of police head count.
Chicago Mayor Rahm Emanuel was forced to close three of the city’s police stations in 2012 due to budget shortfalls. In 2011 Detroit cut police salaries, then laid off 14 percent of the force; police responded by distributing leaflets headlined “Enter Detroit at Your Own Risk.” One of America’s most crime-ridden cities – Camden, N.J. – shut down its entire police force in 2012, migrating instead to a regional model.
For municipal police, a crossroad awaits – downsize and struggle, or downsize and re-invent. Based on results from the first generation of data analytics programs already in the field, the future of law enforcement will, thankfully, be one of re-invention. Data-based solutions and smarter deployment of resources are taking crime prevention to a new level, extending the scope and value of police work beyond traditional physical boundaries, while reducing costs at the same time.
Now is the time to not only catch snipers and criminals before they act, but also to empower our essential public guardians to serve the public good more effectively than ever.
Main photo courtesy of Shutterstock