In this installment of the Innovation of the Month series, we explore how the city of Austin, Texas, in partnership with the University of Texas at Austin, is using video analytics to assess the city’s traffic and road usage patterns to better inform transportation analytics.
MetroLab’s Executive Director Ben Levine spoke with John Clary, Data and Technology Services Senior Supervisor at the Austin Transportation Department (ATD); Jen Duthie, Arterial Management Division Manager at ATD; Joel Meyer, Pedestrian Program Manager at ATD; Natalia Ruiz Juri, Research Lead at University of Texas’s (UT) Center for Transportation Research; and Weijia Xu, Manager for Data Mining and Statistics at UT’s Advanced Computing Center to discuss the project.
Ben Levine: Tell me about Automated Video Analytics for Improved Mobility.
Jen Duthie: The goal of the project is to leverage the city of Austin’s existing traffic monitoring cameras to obtain data on roadway usage and safety. The University of Texas research team has built a tool that uses existing video streams from CCTV cameras to detect, track and query objects such as vehicles and pedestrians. A demonstration can be viewed here and more information on the technical aspects of the tool can be found here.
Weijia Xu: The project team has set up a processing pipeline to stream and process live traffic cameras using computational resources at the Texas Advanced Computing Center (TACC). Both intermediate video recordings and processed results of video files are stored and managed at TACC. The pipeline can assist the city on a variety ad hoc analytics approaches, such as getting traffic summaries and insights of road usage patterns over a specific time and location. The processed results can also be reused for future analysis.
Natalia Ruiz Juri: This project is different from other available tools in that it uses a two-stage approach. In the first stage, objects in each frame are recognized and stored in structure files, which can be managed and stored with a large-scale data system such as Hadoop. Additional analyses, such as tracking and specific scenario detection, are conducted in the second stage utilizing big data processing framework SPARK. The approach provides flexibility in the use of the data, and potentially a solution to archive large volumes of video data for future studies. Large files may be processed and stored in a database, and efficiently queried for multiple analyses as new needs emerge.
Levine: What was the motivation to address this particular challenge?
Joel Meyer: This project offers the opportunity to use existing city assets to collect information that can help us better understand the experiences of Austin pedestrians so we can make more informed decisions and make walking safer and more enjoyable. Traditionally, transportation planners have relied on time-consuming data collection methods such as field observations and manual counts to gain insights into the behaviors of people walking and interactions between pedestrians and drivers. There is a big need for more and better data to help cities answer questions such as how many people are walking in a given area, where people are crossing the street, and how better pedestrian infrastructure might influence user behavior.
Ruiz Juri: The process of video data analysis often requires significant human effort, and minimizing such effort was an initial motivation for this project. As we better understand the potential of automating the identification of objects, trajectories and activities, we can envision further applications.
Xu: Artificial intelligence (AI) and Internet of Things (IoT) devices are two emerging technologies bringing drastic changes to our everyday life. However, practical challenges remain with adoption of those technologies. IoT and embedded smart devices are usually designed for specific purposes with high initial deployment costs. AI often requires large, labeled data sets and computing resources in order to build a good model. Therefore, the project is also motivated by exploring a practical solution that can utilize existing resources.

Figure: Camera access and processing pipeline overview. Courtesy of the city of Austin.
Levine: How did the partnership between the city, the University of Texas at Austin Center for Transportation Research, and the Texas Advanced Computing Center inform this work?
Duthie: The scope for the project was co-created based on a need that the city has for more and better data about the transportation system. Video analytics is a hot topic right now and partnering with UT allows us to understand this technology and be better prepared to ask the right questions when we’re approached by vendors. Most vendor systems, however, rely on installing new cameras. If UT can help us build an object-detection solution that uses our existing traffic monitoring cameras, then we can save quite a bit of money and obtain data that is crucial for effective planning and operations.
Ruiz Juri: The collaboration with TACC helped our Center for Transportation Research (CTR) better understand the potential of existing AI libraries, which was critical in identifying applications to address the needs of the city. The scope of the project itself is continuously adjusted as part of the collaborative relationship between TACC, CTR and the city of Austin.
Xu: As one of the leading supercomputing institutes for open science in the nation, TACC is also keen to bring in the latest technology advances for practical problems. So TACC appreciates this collaboration opportunity with CTR and the city. We are defining and adapting the goal of the project along the way based on the city's needs so that the project, in addition to driving novel research, can result in useful tools and services for the city.
Levine: How will the findings developed in this project impact city planning and management of the built environment?
Meyer: There are a number of ways that we anticipate using the information collected from this project to improve how we plan for pedestrians. At the most basic level, the data will give us a much better understanding of how many people are currently walking in different areas of the city and will allow us to measure citywide walking trends over time.
The data collected from this project will also provide us with new insights into where people are currently crossing the street and how far out of their way they might be willing to walk to use a safe crossing. This will allow us to be proactive in constructing new crossings in areas where there is demand but not safe crossings. We hope that this type of information will help us identify and prioritize locations where new infrastructure can help reduce risky pedestrian behaviors, such as crossing mid-block on high-speed arterial roads.
Longer term, we are excited about the potential that this type of technology has to help identify where and how often near misses between drivers and pedestrians are occurring so we can implement countermeasures to prevent crashes before they happen.
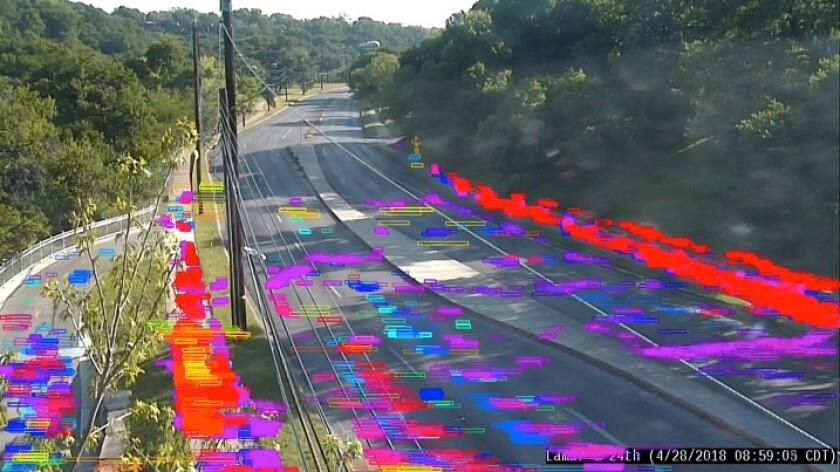

Figure: Visual summaries of pedestrian activity from recordings at Lamar Blvd. & 24th St. including location summary (top) and tracking summary (bottom). Courtesy of the City of Austin.
Levine: What was the most surprising thing you learned during this process?
Meyer: We knew that accurately capturing the movements of people in complex urban environments would present a challenge to our researchers, but I underestimated just how difficult it would be. Avoiding double-counting a person lingering at a bus stop, for example, or counting the number of individuals within a group of people crossing the street, has proven to be very challenging. But we are excited about the progress that our partners at UT have made in tackling these technical hurdles.
Juri: For me, it was surprising to see how difficult it may be, even for a human observer, to estimate safety-related metrics such as near misses or unsafe distances between pedestrians and moving vehicles in a video stream. It's interesting to think that, with proper training, some of these events may be identified automatically. I was also surprised by some examples where the relatively simple algorithms currently implemented were able to detect objects that humans may have missed.
Xu: We have learned a lot about traffic research and city planning along the way. One of the most surprising things is the diversity of different scenarios in real life. We start with a few simple assumptions, which need to be adjusted in order to improve the model to detect those unexpected cases. However, a challenge is the lack of well-curated data and the difficulty of curating data manually.
Levine: You mentioned some of the issues associated with individual identification. That brings up an important topic relevant to so many data collection efforts: privacy. As you integrate data from CCTV cameras, you will also be stringing together peoples’ movements. How are you ensuring residents’ privacy is protected and how are you communicating that issue to the public?
John Clary: The data we’re collecting is traces of movements across a relatively small amount of space — an intersection, for example. We do not store any identifying information other than the path the object took. We have no intention of warehousing the actual video recordings, those will be disposed of once we run the data through the computer vision tool. It’s the output of the computer vision that we’re archiving, which, again, is just the object classification.
Levine: What are the future plans for this project?
Duthie: We are going to start using some of the capabilities that the research team has developed to assist in the planning of new traffic signal locations, and in evaluating the performance of existing signal locations. The current technology will allow us to see where pedestrians are crossing at an intersection, and compare that to the expected crossing locations. We also want to further develop the ability to use this technology to understand the number of people crossing at each intersection, so we can start to understand the demand.
Xu: Although we feel that we have accomplished a lot, many interesting problems remain to be investigated. These include detecting and classifying different pedestrian behaviors, and providing a useful management and analysis interface for data reuse. We are also interested in creating a software tool or cloud service that the city can easily use to understand vehicle and pedestrian movements on the roads.
About MetroLab: MetroLab Network introduces a new model for bringing data, analytics, and innovation to local government: a network of institutionalized, cross-disciplinary partnerships between cities/counties and their universities. Its membership includes more than 35 such partnerships in the United States, ranging from mid-size cities to global metropolises. These city-university partnerships focus on research, development, and deployment of projects that offer technologically- and analytically-based solutions to challenges facing urban areas including: inequality in income, health, mobility, security and opportunity; aging infrastructure; and environmental sustainability and resiliency. MetroLab was launched as part of the White House’s 2015 Smart Cities Initiative. Learn more at www.metrolabnetwork.org or on Twitter @metrolabnetwork.